|
Anja Butter
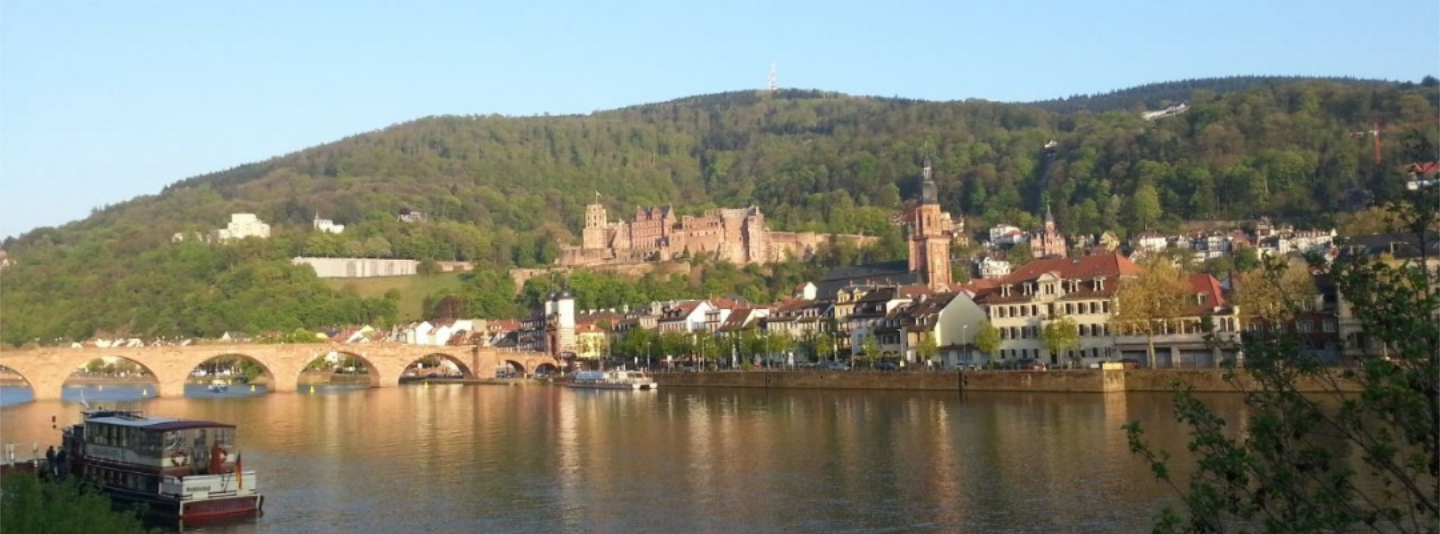
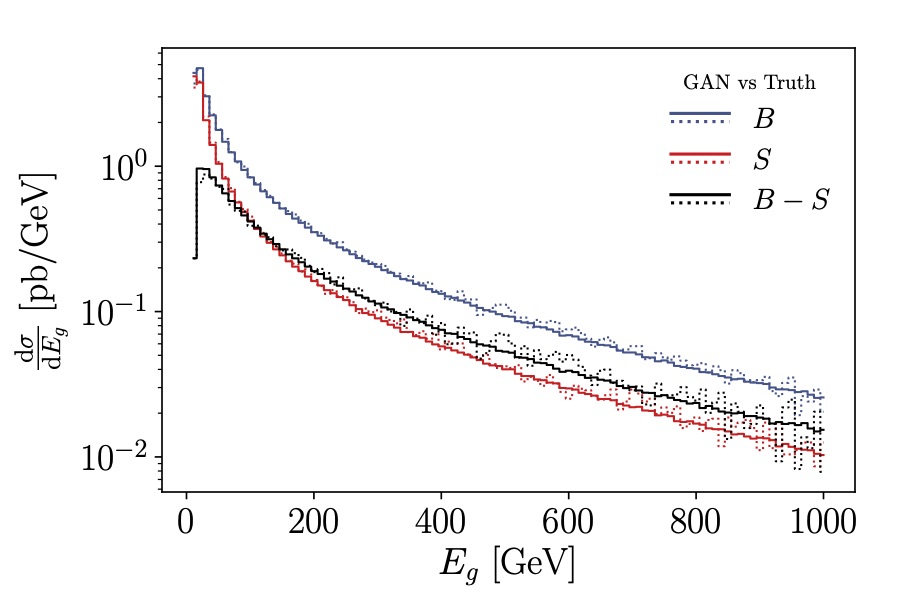
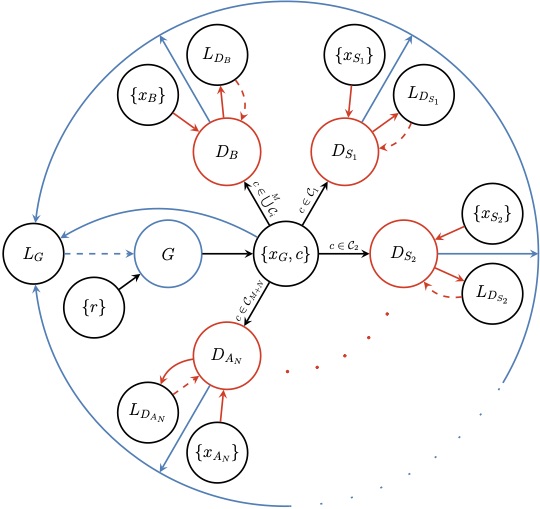
Generative networks
A very promising machine learning application is the use of generative neural networks for event simulation. We have shown that generative adversarial networks can learn the full underlying phase space distribution of events to generate independent new samples.
The flexibility of neural networks allows us to construct tools for previously unattempted tasks. For instance the subtraction GAN shown here learns to populate the phase space between two distributions represented by samples.
Global analyses of dark matter Global analyses of dark matter models allow us to connect measurements from collider, direct and indirect detection measurements. The right hand plot shows regions of the NMSSM (next to minimal supersymmetric extension of the Standard Model) parameter space that are in agreement with limits from direct detection and the measured relic density. Color coded is the resulting invisible branching ratio of the Higgs. We see that there are many regions that would lead to an enhanced branching ratio, making it a promising signature to look for new physics.
Contact
Mail:
Phone: +49 6221 54 9406
Institut für Theoretische Physik
Universität Heidelberg
Philosophenweg 16
D-69120 Heidelberg, Germany