|
Welcome!
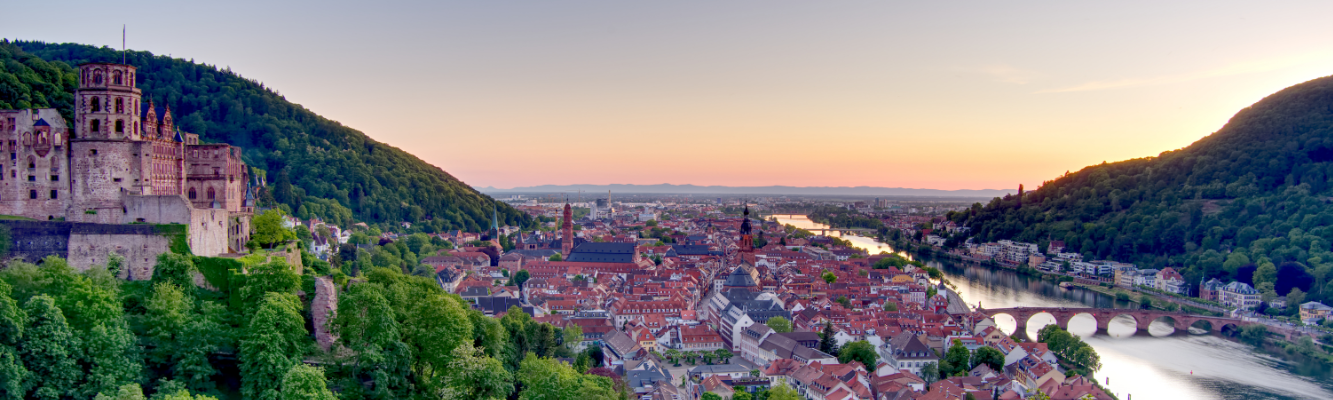
Hello, my name is Lorenz Vogel. I am a final-year Master's student in physics at Heidelberg University, specializing in high-energy physics (HEP) and machine learning (ML). Concepts and tools from data science (especially modern ML) really have great potential to transform particle-physics research.
My fascination with the intersection of (particle) physics and ML already began during my Bachelor's studies in physics at Heidelberg University. I have done my Bachelor's thesis in the LHC Physics and New Particles group at the Institute for Theoretical Physics (ITP) on applying self-supervised contrastive representation learning to particle jets (i.e. narrow cones of particles produced in high-energy particle collisions). Collider searches face the challenge of defining a data representation such that physical symmetries are manifest, the discriminating features are retained, and the choice of representation is new-physics agnostic. Symmetries are the most basic structure in physics and if we want to use ML successfully, we need to embrace these symmetries. To this end, we implemented an ML-training procedure (using a transformer-encoder network) that ensures that the learned jet-representation space is invariant to pre-defined theory-driven symmetries, while still retaining discriminative power for downstream tasks such as jet tagging/classification.
In addition to the Master’s curriculum I am still actively involved in the Heidelberg HEP-ML research group, working on ML projects related to particle physics. In the first project following my Bachelor's thesis, we studied the resilience and generalization errors of ML-based jet taggers when training and testing these classification networks on different particle-physics simulations. Employing a Bayesian neural network (BNN) architecture allowed us to track the stability of the training and to identify training-related uncertainties.
Uncertainty-aware neural networks are extremely powerful numerical tools for high-energy physics, which are not black boxes but instead offer a lot of control and pave the way to interpretable and explainable AI. Therefore, we recently explored the use of uncertainty-aware regression networks (BNNs and repulsive ensembles) for the multi-dimensional calibration of the calorimeter signals in the ATLAS experiment at CERN's Large Hadron Collider (LHC). This (ongoing) collaboration with our experimental colleagues at ATLAS has shown me that the future of LHC research is being inspired and shaped by data science as a new common language of particle experiment and theory.
Contact
Lorenz Vogel, B.Sc.
Email:
Institut für Theoretische Physik
Universität Heidelberg
Philosophenweg 12
69120 Heidelberg, Germany